Hi guys,
I'm pretty much a newbie to SBR, trying to understand how this stuff works. I understand probability/statistics pretty well. It seems to me that you can understand SBR pretty well through Bayesian statistics.
Let's focus on spread betting. The basic idea is that you want to predict as accurately as possible (before the game starts) what the final spread will be. If you had some magical genie that would tell you the exact spread beforehand, life would be great, you'd win every bet.
Of course, this is probably too much to ask for. Suppose instead you had a slightly weaker genie. He cannot predict the future, but instead he can build 100,000 (or some other suitably large number, say 1 billion if you think 100K is too small) perfect copies of the real world, and then play tomorrow's game in each simulated world.
You wouldn't know the final spread for tomorrow's game, but instead you'd have a probability distribution describing it. For simplicity's sake, lets say that the 100K margins the genie gave you are very well approximated by a normal distribution (http://en.wikipedia.org/wiki/Normal_distribution) with say mean -5 and standard deviation 8.
In other words, from the 100k simulations the genie gave you, you feel that the true spread should be -5.
If the Pinnacle spread for the game were say -2, you could then calculate (or consult a Z-scores table, for example) that the probability of the favorite beating the spread is roughly 64.6%.
So in other words, this would be a highly, highly favorable bet for you....you'd beat the spread 64.6% of the time.
Of course, all of this is highly dependent on two factors:
1) How far away is the Pinnacle spread from the "Average" spread the genie gives you? For example, if the Pinnacle spread were changed from -2 to -5 in the example I did above, then you'd have absolutely no advantage...you'd only beat the spread 50% of the time.
2) How big the standard deviation of your genie's estimate. If the standard distribution changed from 8 to say 100 in the above example, then your chance of beating the spread drops down to 51.197% (you can get this value yourself by using a Z-score calculator.)
Does the above conceptually make sense, as a way to understand sports betting conceptually? Of course, you can make this simple setup more precise by progressively weakening the power of your genie. But I'm just trying to figure out if I have a high-level understanding of what it is going on here (at least from a Bayesian sort of perspective.)
Thanks in advance for any clarification/comments.
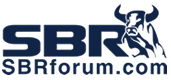