Action Points To Percentage
Like many, I use action points to help determine the strength of models. But the problem with action
points is that you can't add them all up and divide them by the number of games, because 3 pts has
a different value depending on whether the total is 36 or 47.
So I tried to define a calculation to translate action points into percentages by recalibrating the score
into what it would have been if the game had ended exactly on the game total.
Example. Line: Fave -7 with 47 total (inherent score 27-20, with 7 pts being 14.9% of the total).
Result: Dog wins 23-20. Translated to 47 pts that score would be 25.14 - 21.86 (total 47).
So the dog beat the favorite by 3.28 pts against the 47 total, or 7.0%.
Am I correct to reason that the dog beat the spread not just by 10 action points, but by 21.9%? (the
dog covered the 14.9% plus an extra 7.0%). In this example, the 21.9% advantage would translate
as 60.95 % - 39.05%.
If the same +7 dog had lost the game 20-26 (20.43-26.57) the ATS win would have an action points
to percentage number of 4.55% with a distribution of 52.275% - 47.725%.
======================================== =============================
ATS And APTP (Action Points To Percentage)
Hypothesis. If action points can be used to determine the strength of a model in a more reliable way
than just the ATS %, then action points to percentage could, possibly, give a (better) read on the
influence of luck.
The ATS % and the APTP numbers are not the same, but they do point in the same direction in that
they both qualify the strength of a model. Therefore, if a long term 57% ATS model is accompanied
by a long term 60% APTP number, the influence of luck would have been less than if a 57% ATS
model were accompanied by a 54% APTP number. If this reasoning is accurate, or going in the right
direction, then one may be able to identify when a model is over or underperforming, and adjust bet
size accordingly.
Basically, one would have two graph lines for every model, and I believe those two may be better
than one. Especially in fields of smaller sample sizes, such as sports betting, this could be a useful
approach.
======================================== =============================
Thoughts?
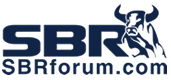